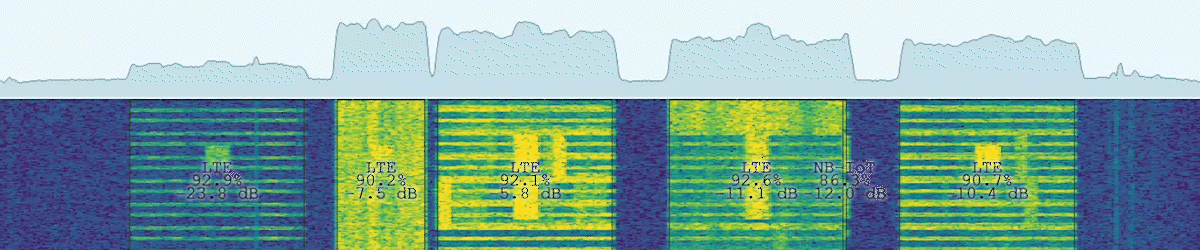
An Epic Traffic Jam
Monitoring and managing radio frequency (RF) usage is vital. It allows the RF spectrum – a fixed resource – to be shared and optimized to support technology advances that deliver ever-faster wireless data speeds, increased network coverage, and support for advanced electromagnetic domain operations.
To say modern RF spectrum monitoring is complex is an understatement. Industries ranging from telecommunications to airspace safety are all struggling to monitor a landscape of crowded, dynamic, and intertwined RF signals. Telecommunications providers must manage increasingly crowded spectrum to mitigate interference and maximize network performance. Defense and security teams face adversaries who cleverly disguise their radio emissions to avoid detection. In civilian airspace, the rise of drones adds yet another layer – detecting a rogue drone’s control signal is like finding a whisper in a shouting crowd. Meanwhile, emerging 5G/6G systems introduce new waveforms and frequency bands that strain traditional network monitoring tools.
In this crowded RF environment, legacy spectrum analyzers and manual monitoring cannot keep up. Signals hop frequencies, hide under noise, or appear only fleetingly. A new approach to RF awareness is needed—one that moves faster and smarter than human analysts or threshold-based detectors.
RF Spectrum Monitoring 2.0
Artificial intelligence (AI) is a game-changer for RF spectrum monitoring. These new capabilities allow operations teams to sift through gigabytes of spectrum data in real-time at the edge and only flag what matters. But, to be effective, AI models need the right platform. A platform that can listen to the radio spectrum and think simultaneously, preferably on the network’s edge, and use AI models to deliver low-latency intelligence.
Deepwave and DeepSig – together – make RF Spectrum Monitoring 2.0 a reality. These best-in-class technologies offer simple deployments and an ‘it-just-works’ user experience, which allows AI scientists, engineers, and product teams to focus on RF insights instead of IT administration.
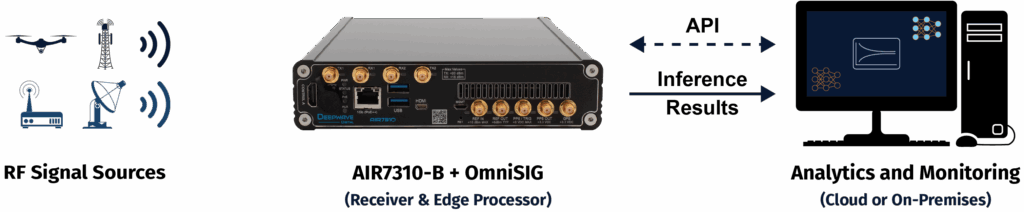
OmniSIG® Engine and Studio
OmniSIG Engine is the runtime AI classifier for RF signals. It ingests raw signal data and uses AI to identify and categorize signals in real time, including known emitters and previously unseen anomalies. Engine can run classification models across a wide frequency range and operates efficiently on embedded edge computing.
OmniSIG Studio speeds AI data preparation and yields high-confidence models that can be deployed with minimal data and time. Studio’s no-code interface for training new signal classification models is designed around RF inference model development. The platform allows RF-savvy analysts the ability to label, annotate, and curate relevant datasets, such as commercial drone telemetry, radar, or communications waveforms, and train new models quickly using an intuitive spectrogram interface. This enables operations teams to quickly develop and deploy classifiers for protocols like Ocusync or MAVLink, which may include wideband OFDM video downlinks and narrowband frequency-hopping control signals.
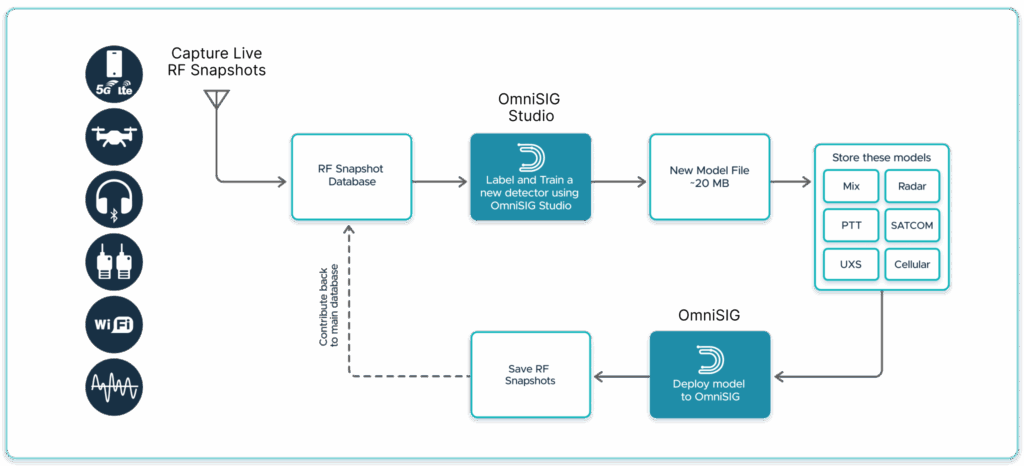
AirStack Core and AIR-T Embedded Series
Deepwave’s AIR-T is the world’s first GPU-based software-defined radio (SDR) designed specifically for RF and wireless deep learning applications. The AIR-T significantly reduces the complexities of RF software, hardware, and systems integration. Our AIR-T system is designed to turn on and go.
The AIR7310-B is Deepwave’s enterprise intelligence platform. This SDR is purpose-built for real-time AI inference at the edge. Key components include a wideband RF front-end (2×2 MIMO, 300 MHz to 6 GHz), an AMD FPGA for real-time signal processing, and an NVIDIA Jetson Orin NX for GPU-accelerated AI processing—all within a compact, rugged housing. This enterprise RF intelligence platform also features preconfigured RF chains with programmable gain and filtering, integrated GPS timing, and 2TB NVMe storage for on-site data collection.
AirStack Core is the centerpiece of Deepwave’s platform. It allows the AIR-T edge platform to deliver AI-generated RF intelligence. Core’s unique capabilities allow RF signals to be analyzed at the edge, so only AI-generated results are shared with connected IT systems. Sending only AI model results means the data output from the AIR-T is significantly reduced and, more importantly, low-latency intelligence is delivered so autonomous systems and operations platforms can take actions.
AirStack Core includes AIR-T device drivers, APIs, CUDA support, and integration with ML frameworks like Triton Inference Server and TensorRT. This software platform also allows users to interface directly with the RF, GPU, and FPGA resources and supports containerized workflows for fast deployment.
AirStack Core’s features enable:
- Accelerated Computing – Based on Ubuntu + NVIDIA JetPack. Enables zero-copy pipeline from RF to AI inference.
- MLOps and Edge Management – Remote management and model updates.
Power of the Combined System: AIR-T + AirStack + OmniSIG
Deepwave and DeepSig are excited to offer a turn-key Machine Learning Operations (MLOps) pipeline for RF AI. An integrated OmniSIG, AIR-T, and AirStack solution is easy to configure and deploy, so operations teams can focus on generating and using RF intelligence.
Operators can deliver rapid MLOps development cycles on a Deepwave and DeepSig solution: creating AI-ready data by collecting snapshots of target RF activity using the AIR7310-B, labeling and annotating control and data bursts (such as drone uplinks and video downlinks), training a deep learning model, and CI/CD features to deploy a model to AIR-T hardware for inference immediately. These RF AI models—typically just a few megabytes in size—can be pushed over low-bandwidth links and activated instantly, turning a deployed sensor into a tailored classifier in hours. Integration with data pipelines allows detections to be streamed to dashboards, databases, or automation systems using ZeroMQ, websockets, or file exports.
Deepwave and DeepSig are tightly integrated to power end-to-end RF MLOps:
- High-confidence AI results: OmniSIG Engine delivers confident detections even in low SNR and dense environments—ideal for drone signals using Ocusync, MAVLink, or frequency-hopping protocols.
- Real-time edge inference: Wideband RF data flows directly into the GPU for classification without latency overhead.
- Custom model deployments: Trained in OmniSIG Studio and deployed in seconds via AirStack Core.
- Scalable edge field deployments: Units can be managed remotely, updated over-the-air, and deployed in fleets.
Conclusion
In today’s complex RF environment, Deepwave’s AIR-T platform and AirStack software, paired with DeepSig’s OmniSIG Engine and Studio, offer a robust, edge-deployable solution for delivering RF signal intelligence. From tactical operations to automated 5G interference monitoring, this plug-and-go Deepwave and DeepSig solution enables high-performance AI inference where it matters most. Deepwave and DeepSig – together – make RF Spectrum Monitoring 2.0 a reality.
How to Purchase
Ready to deploy edge AI for RF signal classification? Let us help you transform your RF operations with intelligent, easy-to-deploy solutions. Contact Deepwave today.